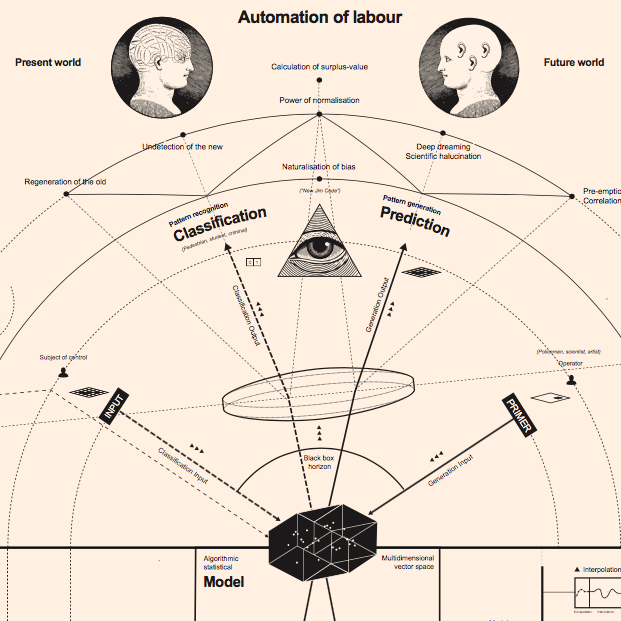
O nooscópio de Pasquinelli e Joler
PASQUINELLI, Matteo; JOLER, Vladan.2020. The Nooscope manifested: artificial intelligence as instrument of knowledge extractivism. KIM research group (Karlsruhe University of Arts and Design) and Share Lab (Novi Sad), 1 May 2020 (preprint forthcoming for AI and Society). https://nooscope.ai
CARTOGRAFANDO OS LIMITES DA IA
The Nooscope is a cartography of the limits of artificial intelligence (Pasquinelli e Joler 2020:2)
MAPAS (debater perspectivas parciais)
Any map is a partial perspective, a way to provoke debate. (Pasquinelli e Joler 2020:2)
A MISTIFICAÇÃO DA INTELIGÊNCIA ARTIFICIAL DO SÉCULO XXI (exploração econômica do conhecimento fantasiada de automagia)
In the expression ‘artificial intelligence’ the adjective ‘artificial’ carries the myth of the technology’s autonomy: it hints to caricatural ‘alien minds’ that self-reproduce in silico but, actually, mystifies two processes of proper alienation: the growing geopolitical autonomy of hi-tech companies and the invisibilization of workers’ autonomy worldwide. The modern project to mechanise human reason has clearly mutated, in the 21st century, into a corporate regime of knowledge extractivism and epistemic colonialism. (Pasquinelli e Joler 2020:2)
NOOSCÓPIO (aprendizado de máquina como mecanismo para magnificar o conhecimento)
machine learning is just a Nooscope, an instrument to see and navigate the space of knowledge (from the Greek skopein ‘to examine, look’ and noos ‘knowledge’). (Pasquinelli e Joler 2020:2)
BIAS NO APRENDIZADO DE MÁQUINA (alucinação estatística como o novo regime de verdade-prova-normatividade-racionalidade)
In the same way that the lenses of microscopes and telescopes are never perfectly curvilinear and smooth, the logical lenses of machine learning embody faults and biases. To understand machine learning and register its impact on society is to study the degree by which social data are diffracted and distorted by these lenses. This is generally known as the debate on bias in AI, but the political implications of the logical form of machine learning are deeper. Machine learning is not bringing a new dark age but one
of diffracted rationality, in which, as it will be shown, an episteme of causation is replaced by one of automated correlations. More in general, AI is a new regime of truth, scientific proof, social normativity and rationality, which often does take the shape of a statistical hallucination. (Pasquinelli e Joler 2020:2)
AI is not a monolithic paradigm of rationality but a spurious architecture made of adapting techniques and tricks. Besides, the limits of AI are not simply technical but are imbricated with human bias. (Pasquinelli e Joler 2020:3)
IA : MÁQUINA A VAPOR :: TEORIA DO APRENDIZADO : TERMODINÂNICA
AI is now at the same stage as when the steam engine was invented, before the laws of thermodynamics necessary to explain and control its inner workings, had been discovered. Similarly, today, there are efficient neural networks for image recognition, but there is no theory of learning to explain why they work so well and how they fail so badly. (Pasquinelli e Joler 2020:3)
APRENDIZADO DE MÁQUINA (dataset+algoritmo+modelo)
As an instrument of knowledge, machine learning is composed of an object to be observed (training dataset), an instrument of observation (learning algorithm) and a final representation (statistical model). (Pasquinelli e Joler 2020:3)
BIAS HISTÓRICO
Historical bias (or world bias) is already apparent in society before technological intervention. Nonetheless, the naturalisation of such bias, that is the silent integration of inequality into an apparently neutral technology is by itself harmful. (Pasquinelli e Joler 2020:4)
BIAS DO DATASET
Dataset bias is introduced through the preparation of training data by human operators. The most delicate part of the process is data labelling, in which old and conservative taxonomies can cause a distorted view of the world, misrepresenting social diversities and exacerbating social hierarchies (Pasquinelli e Joler 2020:4)
BIAS O ALGORITMO
Algorithmic bias (also known as machine bias, statistical bias or model bias, to which the Nooscope diagram gives particular attention) is the further amplification of historical bias and dataset bias by machine learning algorithms. […] Since ancient times, algorithms have been procedures of an economic nature, designed to achieve a result in the shortest number of steps consuming the least amount of resources: space, time, energy and labour. 13 The arms race of AI companies is, still today, concerned with finding the simplest and fastest algorithms with which to capitalise data. If information compression produces the maximum rate of profit in corporate AI, from the societal point of view, it produces discrimination and the loss of cultural diversity. (Pasquinelli e Joler 2020:4)
O PROBLEMA-EFEITO CAIXA-PRETA
The black box effect is an actual issue of deep neural networks (which filter information so much that their chain of reasoning cannot be reversed) but has become a generic pretext for the opinion that AI systems are not just inscrutable and opaque, but even ‘alien’ and out of control. (Pasquinelli e Joler 2020:4)
A ASCENSÃO DOS MONOPÓIOS DE IA DO SÉCULO XXI
Mass digitalisation, which expanded with the Internet in the 1990s and escalated with datacentres in the 2000s, has made available vast resources of data that, for the first time in history, are free and unregulated. A regime of knowledge extractivism (then known as Big Data) gradually employed efficient algorithmsto extract ‘intelligence’ from these open sources of data, mainly for the purpose of predicting consumer behaviours and selling ads. The knowledge economy morphed into a novel form of capitalism, called cognitive capitalism and then surveillance capitalism, by different authors. It was the Internet information overflow, vast datacentres, faster microprocessors and algorithms for data compression that laid the groundwork for the rise of AI monopolies in the 21st century. (Pasquinelli e Joler 2020:5)
TUDO COMEÇA NA BASE DE DADOS DE TREINAMENTO (ação humana fundacional)
Data is the first source of value and intelligence. Algorithms are second; they are the machines that compute such value and intelligence into a model. However, training data are never raw, independent and unbiased (they are already themselves ‘algorithmic’). The carving, formatting and editing of training datasets is a laborious and delicate undertaking, which is probably more significant for the final results than the technical parameters that control the learning algorithm. The act of selecting one data source rather than another is the profound mark of human intervention into the domain of the ‘artificial’ minds. (Pasquinelli e Joler 2020:5)
CATEGORIZAÇÕES PARCIAIS
The semiotic process of assigning a name or a category to a picture is never impartial; this action leaves another deep human imprint on the final result of machine cognition. (Pasquinelli e Joler 2020:5)
Machine intelligence is trained on vast datasets that are accumulated in ways neither technically neutral nor socially impartial. Raw data does not exist, as it is dependent on human labour, personal data, and social behaviours that accrue over long periods, through extended networks and controversial taxonomies. (Pasquinelli e Joler 2020:5)
O REFLUXO DA IA SOBRE A CULTURA DIGITAL
The voracious data extractivism of AI has caused an unforeseeable backlash on digital culture: in the early 2000s, Lawrence Lessig could not predict that the large repository of online images credited by Creative Commons licenses would a decade later become an unregulated resource for face recognition surveillance technologies. (Pasquinelli e Joler 2020:6)
2012-2019
If 2012 was the year in which the Deep Learning revolution began, 2019 was the year in which its sources were discovered to be vulnerable and corrupted. (Pasquinelli e Joler 2020:6)
IA = RECONHECIMENTO DE PADRÃO
[T]he Nooscope diagram exposes the skeleton of the AI black box and shows that AI is not a thinking automaton but an algorithm that performs pattern recognition. (Pasquinelli e Joler 2020:7)
PERCEPTRON
The archetype machine for pattern recognition is Frank Rosenblatt’s Perceptron. (Pasquinelli e Joler 2020:7)
A NOVA RAZÃO DO MUNDO (extração-reconhecimento-geração automática de padrões)
Regardless of their complexity, from the numerical perspective of machine learning, notions such as image, movement, form, style, and ethical decision can all be described as statistical distributions of pattern. In this sense, pattern recognition has truly become a new cultural technique that is used in various fields. For explanatory purposes, the Nooscope is described as a machine that operates on three modalities: training, classification, and prediction. In more intuitive terms, these modalities can be called: pattern extraction, pattern recognition, and pattern generation. (Pasquinelli e Joler 2020:7)
A DISTRIBUIÇÃO ESTATÍSTICA DE UM PADRÃO
The business of these neural networks, however, was to calculate a statistical inference. What a neural network computes, is not an exact pattern but the statistical distribution of a pattern. (Pasquinelli e Joler 2020:7)
MODELO ESTATÍSTICO ALGORÍTMICO
Algorithm is the name of a process, whereby a machine performs a calculation. The product of such machine processes is a statistical model (more accurately termed an ‘algorithmic statistical model’). (Pasquinelli e Joler 2020:8)
APRENDIZADO = ALCANÇAR “BOA REPRESENTAÇÃO”
(Pasquinelli e Joler 2020:)
MODELOS SÃO ERRADOS, MAS ÚTEIS
‘All models are wrong, but some are useful’ — the canonical dictum of the British statistician George Box has long encapsulated the logical limitations of statistics and machine learning. (Pasquinelli e Joler 2020:10)
O MAPA E O TERRITÓRIO
AI is a heavily compressed and distorted map of the territory and that this map, like many forms of automation, is not open to community negotiation. AI is a map of the territory without community access and community consent. (Pasquinelli e Joler 2020:10)
RECONHECIMENTO DE IMAGEM-PADRÕES (trabalho da percepção)
image recognition (the basic form of the labour of perception, which has been codified and automated as pattern recognition) (Pasquinelli e Joler 2020:10)
APRENDIZADO DE MÁQUINA (mapa estatístico)
Machine
learning is a term that, as much as ‘AI’, anthropomorphizes a piece of technology: machine learning learns nothing in the proper sense of the word, as a human does; machine learning simply maps a statistical distribution of numerical values and draws a mathematical function that hopefully approximates human comprehension. That being said, machine learning can, for this reason, cast new light on the ways in which humans comprehend. (Pasquinelli e Joler 2020:11)
APRENDIZADO DE MÁQUINA como MINIMIZAÇÃO MATEMÁTICA
As Dan McQuillian aptly puts it: ‘There is no intelligence in artificial intelligence, nor does it learn, even though its technical name is machine learning, it is simply mathematical minimization.’ (Pasquinelli e Joler 2020:11)
APROXIMAÇÃO À FORÇA BRUTA
. The shape of the correlation function between input x and output y is calculated algorithmically, step by step, through tiresome mechanical processes of gradual adjustment (like gradient descent, for instance) that are equivalent to the differential calculus of Leibniz and Newton. Neural networks are said to be among the most efficient algorithms because these differential methods can approximate the shape of any function given enough layers of neurons and abundant computing resources. Brute-force gradual approximation of a function is the core feature of today’s AI, and only from this perspective can one understand its potentialities and limitations — particularly its escalating carbon footprint (the training of deep neural networks requires exorbitant amounts of energy because of gradient descent and similar training algorithms that operate on the basis of continuous infinitesimal adjustments).3 (Pasquinelli e Joler 2020:11)
ESPAÇOS MULTIDIMENSIONAIS DE DADOS
The notions of data fitting, overfitting, underfitting, interpolation and extrapolation can be easily visualised in two dimensions, but statistical models usually operate along multidimensional spaces of data. (Pasquinelli e Joler 2020:12)
O TRUQUE DA REDUÇÃO DIMENSIONAL-CATEGORIAL
AI is still a history of hacks and tricks rather than mystical intuitions. For example, one trick of information compression is dimensionality reduction, which is used to avoid the Curse of Dimensionality, that is the exponential growth of the variety of features in the vector space. […] Dimensionality reduction can be used to cluster word meanings (such as in the model word2vec) but can also lead to category reduction, which can have an impact on the representation of social diversity. Dimensionality reduction can shrink taxonomies and introduce bias, further normalising world diversity and obliterating unique identities. (Pasquinelli e Joler 2020:13)
CLASSIFICAR E PREVER (vigiar e governar)
Classification is known as pattern recognition, while prediction can be defined also as pattern generation. A new pattern is recognised or generated by interrogating the inner core of the statistical model. (Pasquinelli e Joler 2020:13)
Machine learning classification and prediction are becoming ubiquitous techniques that constitute new forms of surveillance and governance. […] The hubris of automated classification has caused the revival of reactionary Lombrosian techniques that were thought to have been consigned to history, techniques such as Automatic Gender Recognition (AGR) (Pasquinelli e Joler 2020:14)
PREVER (reduzir o futuro-desconhecido ao passado-conhecido)
Machine learning prediction is used to project future trends and behaviours according to past ones, that is to complete a piece of information knowing only a portion of it. In the prediction modality, a small sample of input data (a primer) is used to predict the missing part of the information following once again the statistical distribution of the model (this could be the part of a numerical graph oriented toward the future or the missing part of an image or audio file). (Pasquinelli e Joler 2020:13)
O TURCO MECÂNICO
An artwork that is said to be created by AI always hides a
human operator, who has applied the generative modality of a neural network trained on a specific dataset. (Pasquinelli e Joler 2020:14)
GOOGLE DEEP DREAM (inconsciente maquímico da IA)
In DeepDream first experiments, bird feathers and dog
eyes started to emerge everywhere as dog breeds and bird species are vastly overrepresented in ImageNet. It was also discovered that the category ‘dumbbell’ was learnt with a surreal human arm always attached to it. Proof that many other categories of ImageNet are misrepresented. (Pasquinelli e Joler 2020:14)
O PODER NORMATIVO DA IA NO SÉCULO XXI
The normative power of AI in the 21st century has to be scrutinised in these epistemic terms: what does it mean to frame collective knowledge as patterns, and what does it mean to draw vector spaces and statistical distributions of social behaviours? (Pasquinelli e Joler 2020:15)
AI easily extends the ‘power of normalisation’ of modern institutions, among others bureaucracy, medicine and statistics (originally, the numerical knowledge possessed by the state about its population) that passes now into the hands of AI corporations. The institutional norm has become a computational one: the classification of the subject, of bodies and behaviours, seems no longer to be an affair for public registers, but instead for algorithms and datacentres. (Pasquinelli e Joler 2020:15)
BIAS ANTI-NOVIDADE-DESVIO
A gap, a friction, a conflict, however, always persists between AI statistical models and the human subject that is supposed to be measured and controlled. This logical gap between AI statistical models and society is usually debated as bias. It has been extensively demonstrated how face recognition misrepresents social minorities and how black neighbourhoods, for instance, are bypassed by AI-driven logistics and delivery service. If gender, race and class discriminations are amplified by AI algorithms, this is also part of a larger problem of discrimination and normalisation at the logical core of machine learning. The logical and political limitation of AI is the technology’s difficulty in the recognition and prediction of a new event. How is machine learning dealing with a truly unique anomaly, an uncommon social behaviour, an innovative act of disruption? (Pasquinelli e Joler 2020:15-6)
A logical limit of machine learning classification, or pattern recognition, is the inability to recognise a unique anomaly that appears for the first time, such as a new metaphor in poetry, a new joke in everyday conversation, or an unusual obstacle (a pedestrian? a plastic bag?) on the road scenario. The undetection of the new (something that has never ‘been seen’ by a model and therefore never classified before in a known category) is a particularly hazardous problem for self-driving cars and one that has already caused fatalities. Machine learning prediction, or pattern generation, show similar faults in the guessing of future trends and behaviours. As a technique of information compression, machine learning automates the dictatorship of the past, of past taxonomies and behavioural patterns, over the present. This problem can be termed the regeneration of the old — the application of a homogenous space-time view that restrains the possibility of a new historical event. (Pasquinelli e Joler 2020:16)
APRENDIZADO DE MÁQUINA como ARTE ESTATÍSTICA
The ‘creativity’ of machine learning is limited to the detection of styles from the training data and then random improvisation within these styles. In other words, machine learning can explore and improvise only within the logical boundaries that are set by the training data. For all these issues, and its degree of information compression, it would be more accurate to term machine learning art as statistical art. (Pasquinelli e Joler 2020:16)
CAUSA =/= CORRELAÇÃO
Another unspoken bug of machine learning is that the statistical correlation between two phenomena is often adopted to explain causation from one to the other. In statistics, it is commonly understood that correlation does not imply causation, meaning that a statistical coincidence alone is not sufficient to demonstrate causation. […] Superficially mining data, machine learning can construct any arbitrary correlation that is then perceived as real. I (Pasquinelli e Joler 2020:16)
According to Dan McQuillan, when machine learning is applied to society in this way, it turns into a biopolitical apparatus of preemption, that produces subjectivities which can subsequently be criminalized. Ultimately, machine learning obsessed with ‘curve fitting’ imposes a statistical culture and replaces the traditional episteme of causation (and political accountability) with one of correlations blindly driven by the automation of decision making. (Pasquinelli e Joler 2020:16)
PROCESSOS DE SUBJETIVAÇÃO (emancipação do datacentrismo)
How to emancipate ourselves from a data-centric view of the world? It is time to realise that it is not the statistical model that constructs the subject, but rather the subject that structures the statistical model. Internalist and externalist studies of AI have to blur: subjectivities make the mathematics of control from within, not from without. To second what Guattari once said of machines in general, machine intelligence too is constituted of ‘hyper-developed and hyperconcentrated forms of certain aspects of human subjectivity.’ (Pasquinelli e Joler 2020:)
SABOTAGEM (ataques, hacks, exploits)
Adversarial attacks exploit blind spots and weak regions in the statistical model of a neural network, usually to fool a classifier and make it perceive something that is not there. […] Adversarial examples are designed knowing what a machine has never seen before. This effect is achieved also by reverse-engineering the statistical model or by polluting the training dataset. (Pasquinelli e Joler 2020:18)
Adversarial attacks remind us of the discrepancy between human and machine perception and that the logical limit of machine learning is also a political one. The logical and ontological boundary of machine learning is the unruly subject or anomalous event that escapes classification and control. The subject of algorithmic control fires back. Adversarial attacks are a way to sabotage the assembly line of machine learning by inventing a virtual obstacle that can set the control apparatus out of joint. An adversarial example is the sabot in the age of AI. (Pasquinelli e Joler 2020:19)
TRABALHO FANTASMA
Pipelines of endless tasks innervate from the Global North into the Global South; crowdsourced platforms of workers from Venezuela, Brazil and Italy, for instance, are crucial in order to teach German self-driving cars ‘how to see.’ Against the idea of alien intelligence at work, it must be stressed that in the whole computing process of AI the human worker has never left the loop, or put more accurately, has never left the assembly line. Mary Gray and Siddharth Suri coined the term ‘ghost work’ for the invisible labour that makes AI appear artificially autonomous. (Pasquinelli e Joler 2020:)
HETEROMAÇÃO
Automation is a myth; because machines, including AI, constantly call for human help, some authors have suggested replacing ‘automation’ with the more accurate term heteromation. Heteromation means that the familiar narrative of AI as perpetuum mobile is possible only thanks to a reserve army of workers. (Pasquinelli e Joler 2020:19)
INTELIGÊNCIA ARTIFICIAL = DIVISÃO DO TRABALHO = AUTOMAÇÃO DO TRABALHO
the origin of machine intelligence is the division of labour and its main purpose is the automation of labour. (Pasquinelli e Joler 2020:19)
O TURCO MECÂNICO DO CONHECIMENTO
The Nooscope’s purpose is to expose the hidden room of the corporate Mechanical Turk and to illuminate the invisible labour of knowledge that makes machine intelligence appear ideologically alive. (Pasquinelli e Joler 2020:20)