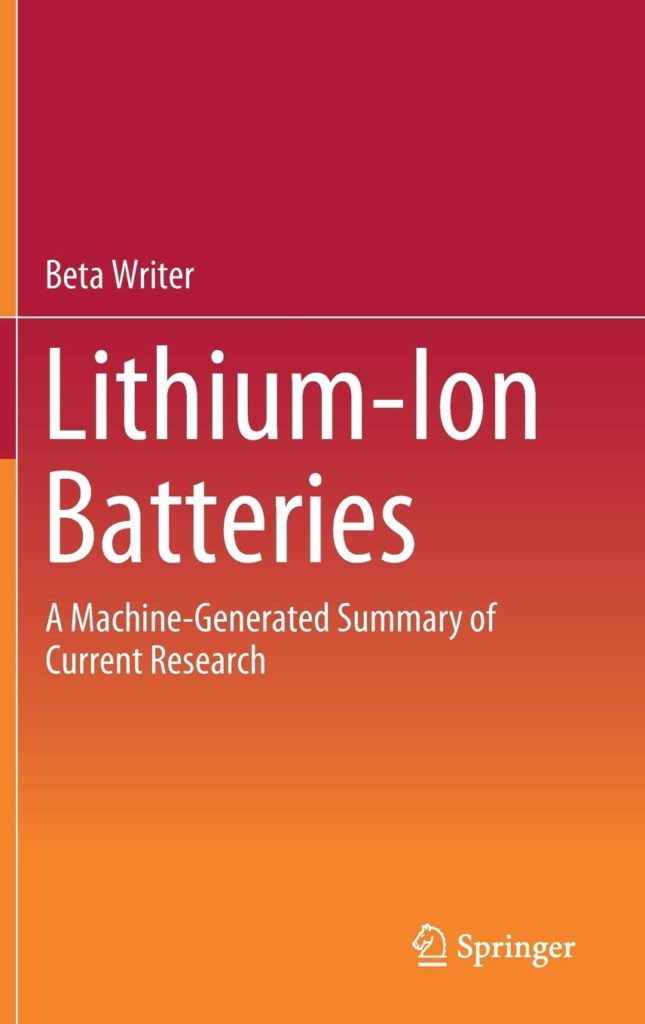
Escrevendo escritores (o caso Beta Writer)
SCHOENENBERGER, Henning. 2019. Introduction. In: Beta Writer. Lithium-ion batteries: a machine-generated summary on current research. Heidelberg: Springer, pp. v-x.
LIVRO “ESCRITO” POR COMPUTADOR
[This book] is the first machine-generated research book. This book […] has the potential to start a new era in scientific publishing. With the exception of this preface it has been created by an algorithm on the basis of a re-combined accumulation and summarization of relevant content in the area of Chemistry and Materials Science. (Schoenenberger 2019:v)
O “PROBLEMA” DO AUTOR
Who is the originator of machine-generated content? Can developers of the algorithms be seen as authors? Or is it the person who starts with the initial input (such as “Lithium-Ion Batteries” as a term) and tunes the various parameters? Is there a designated originator at all? Who decides what a machine is supposed to generate in the first place? Who is accountable for machine-generated content from an ethical point of view? (Schoenenberger 2019:vii)
[W]what does all this mean for the role of the scientific author? We foresee that in future there will be a wide range of options to create content—from entirely human-created content to a variety of blended man-machine text generation to entirely machine-generated text. We do not expect that authors will be replaced by algorithms. On the contrary, we expect that the role of researchers and authors will remain important, but will substantially change as more and more research content is created by algorithms. To a degree, this development is not that different from automation in manufacturing over the past centuries which has often resulted in a decrease of manufacturers and an increase of designers at the same time. Perhaps the future of scientific content creation will show a similar decrease of writers and an increase of text designers or, as Ross Goodwin puts it, writers of writers: “When we teach computers to write, the computers don’t replace us any more than pianos replace pianists—in a certain way, they become our pens, and we become more than writers. We become writers of writers.” (Schoenenberger 2019:ix)
O “PROBLEMA” DO EDITOR
How will the publication of machine-generated content impact our role as a research publisher? (Schoenenberger 2019:viii)
O TEXTO “NÃO FOI EDITADO”
Truly, we have succeeded in developing a first prototype which also shows that there is still a long way to go: the extractive summarization of large text corpora is still imperfect, and paraphrased texts, syntax and phrase association still seem clunky at times. However, we clearly decided not to manually polish or copy-edit any of the texts due to the fact that we want to highlight the current status and remaining boundaries of machine-generated content. (Schoenenberger 2019:viii)
“AINDA” PRECISAMOS DE HUMANOS
[W]e still think that for the foreseeable future we will need a robust human review process for machine-generated text. (Schoenenberger 2019:ix)
AUMENTANDO A “EFICIÊNCIA” DA HUMANIDADE
We do join Zackaray Thoutt’s enthusiasm who indicates that “technology is finally on the cusp of breaking through the barrier between interesting toy projects and legitimate software that can dramatically increase the efficiency of humankind.” (Schoenenberger 2019:x)
QUEM É “PAR” DA MÁQUINA?
The term peer itself indicates a certain inadequacy for machine-generated research content. Who are the peers in this context? Would you as a human reader consider yourself as peer to a machine? And should an expert in a specific research field become an expert of neural networks and Natural Language Processing as well in order to be able to evaluate the quality of a text and the related research? (Schoenenberger 2019:ix)
CHIARCOS, Christian; SCHENK, Niko. 2019. Book generation system pipeline. In: Beta Writer. Lithium-ion batteries: a machine-generated summary on current research. Heidelberg: Springer, pp. x-xxiiii.
ESTRUTURAR O NÃO-ESTRUTURADO
Automatically generating a structured book from a largely unstructured collection of scientific publications poses a great challenge to a computer which we approach with state-of-the-art Natural Language Processing (NLP) and Machine Learning techniques. (Chiarcos e Schenk 2019:x)
ABORDAGEM “CONSERVADORA”
As creators and consumers of scientific publications tend to value correctness over style, we eventually decided for a relatively conservative approach, a workflow based on […] 1. document clustering and ordering, […] 2. extractive summarization, and […] 3. paraphrasing of the generated extracts. (Chiarcos e Schenk 2019:xi)
Guided by subject matter experts on chemistry and social sciences, we eventually went for a conservative approach to book generation, in that as much information is preserved from the original as possible. (Chiarcos e Schenk 2019:xxiii)
HUMAN FEEDBACK – AND HUMAN FEEDBACK ONLY
we designed a workflow according to the premise to preserve as much as possible from the original text—while still producing readable, factually correct, compact, and, of course, novel descriptions. The interested reader may decide to what extent we achieved this goal, but more importantly, let us know where we failed, as it is human feedback—and human feedback only—that can improve the advance of artificial authoring (Chiarcos e Schenk 2019:xi)
PALAVRAS-CHAVE INVESTIGADAS
In the present volume this includes, e.g., any realization of “li-ion battery”, “lithium-ion batteries”, etc. and all occurrences containing “anode” and/or “cathode” as found in either article, chapter, book titles or document meta data. (Chiarcos e Schenk 2019:xiii; nota 3)
APESAR DE ESPECIALISTAS TEREM MEXIDO NO TEXTO, ELES TENTAM ARGUMENTAR QUE ELE AINDA É AUTOMÁTICO…
Even though the structure generation for the manuscript is fully automated, here, a number of parameter values can be set and tuned by the human expert who uses the program, such as the desired number of chapters (i.e., cluster prototypes) and sections, as well as the number of document assignments per section. The result of this process is a structured table of content, i.e., a manuscript skeleton in which pointers to single publications serve as placeholder for the subsequent text. […] At this level, subject matter experts requested the possibility for manual refinement of the automatically generated structure. We permit publications to be moved or exchanged between chapters or sections, or even removed if necessary, for example, if they seem thematically unrelated according to the domain expertise of the editor. We consider the resulting publication nevertheless to be machine-generated, as such measures to refine an existing structure are comparable to interactions between editors of collected volumes and contributing authors, e.g., during the creation of reference works. (Chiarcos e Schenk 2019:xiv-v)
For the present volume, 9 documents have been moved between chapters, and 8 documents were excluded from the final book. Overall, the generated book is based on 151 distinct publications. (Chiarcos e Schenk 2019:xv; nota 5)
Apart from the fully automated text generation module, the human user still has influence on the quality of the text, for example by specifying a list of prohibitive synonym replacements, or by setting the thresholds for the replacements. For compiling this volume, we selected among the aforementioned modules and adjusted their respective threshold in accordance with the feedback from subject matter experts. (Chiarcos e Schenk 2019:xix)
NESTE CASO, NÃO MEXERAM NAS PALAVRAS-CHAVE
Chapter and section headings are represented as a list of automatically generated keywords. Technically, these keywords are the most distinctive linguistic phrases (n-gram features) as obtained as a side-product of the clustering process and are characteristic for a particular chapter/section. Again, human intervention is possible at this stage, for instance, in order to select the most meaningful phrases for the final book. In the present volume, the keywords remained unchanged. (Chiarcos e Schenk 2019:xv)
SUMMARY & CONCLUSION
The summary length (in words and as a proportion of the original text length) is parameterizable by the human editor who uses the system. The conclusion of the book is built in the same way. The introduction produced in this way is conservative in that it reflects the introductions of the input documents selected for the chapter—both in order and content. (Chiarcos e Schenk 2019:xvi)
The summary length has been set to either 270 words or 60% of the original text length— depending on which one was shorter. This combined metric handles the trade-off between too lengthy summaries on the one hand, and summaries which contain almost every sentence of the source, on the other (Chiarcos e Schenk 2019:xvi; nota 7)
COMO COPIAR PARECENDO ORIGINAL?
In order to create text which is not only novel with respect to its arrangement, but also with respect to its formulation, and in order to circumvent issues related to copyright of the original texts, we attempt to reformulate a majority of the sentences as part of the generated book, while trying to preserve their original meaning as best as possible. (Chiarcos e Schenk 2019:xvii)
More than 96% of all sentences were modified by at least one semantic substitution. Sentence compression was kept in a very conservative mode and removed only a small portion of 0.9% of the tokens. In order to acknowledge the original source, every sentence is coupled with the DOI of its source document. In addition, sentences which were not affected by reformulation, synonym replacements, or sentence compression are marked as literal quotes (1.2% of all sentences). (Chiarcos e Schenk 2019:xix)
O LONGO RABO
The nasty little details: Last but not least, we have to mention that a great deal of the errors that we are currently facing are due to specifics of the domain and the data. The interested reader will immediately spot such apparently obvious errors—with rather obvious solutions. This includes, for example, the occasional use of us, ourselves, this paper etc. which refers back to the original publication but is clearly misplaced in the generated book. The solution to these is a simple replacement rule, the challenge in this solution is the sheer number and the distribution of errors that require a domain-specific solution each, sometimes referred to as ‘the long tail’. While we made some efforts to cover such obvious cases, continuous control and refinement of an increasingly elaborate set of repair rules is necessary, and will accompany the subsequent use and development of the Beta Writer. (Chiarcos e Schenk 2019:xxii)
A GRANDE IDEIA
It is to be noted, however, that users would apparently like to scale freely between different degrees of reduction and reformulation, ranging from literal quotes to complete paraphrases. Our implementation does not provide such an interface, but developing such a tool may be a direction for future extensions. (Chiarcos e Schenk 2019:xix)
Getting the human in the loop: Error correction can potentially also be covered by a human expert—or, in a book production workflow, as part of copyediting. But even beyond this level of manual meddling with the machine-generated manuscript, a clear, and somewhat unexpected result of our internal discussions with subject matter experts on chemistry and social sciences was that editors would like to maintain a certain level of control. At the moment, the system remains a blackbox to its users, and we manually adjust parameters or (de)select modules according to the feedback we get about the generated text, then re-generate, etc. At the same time, it is impossible to optimize against a gold standard—because such data does not exist. One solution is to provide a user interface that allows a user to switch parameters on the fly and see and evaluate the modifications obtained by this and thus optimize the machine-generated text according to personal preferences, and—also depending on the feedback we elicit on this volume—developing such an interface is a priority for the immediate future. (Chiarcos e Schenk 2019:xxii)
Another technical challenge that we identified during the creation of this book was that human users aim to remain in control. While an automatically generated book may be a dream come true for providers and consumers of scientific publications (and a nightmare to peer review), advanced interfaces to help users to guide the algorithm, to adjust parameters and to compare their outcomes seem to be necessary to ensure both standards of scientific quality and correctness. (Chiarcos e Schenk 2019:xxiii)